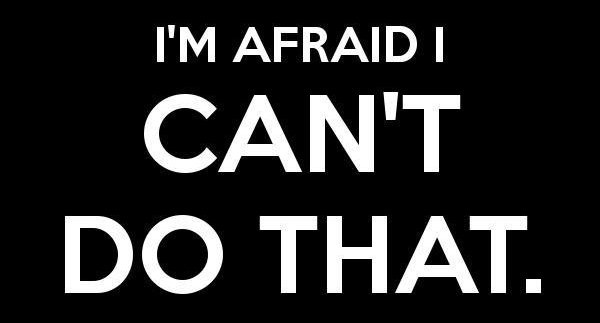
You may already know a lot about Artificial Intelligence (AI), Machine Learning (ML), Deep Learning or even what some vendors call Cognitive Computing. Or maybe you are still trying to understand the nuances and differences between each term, and how they relate to each other.
Either way, it’s easy to be seduced by the “black magic” of technology that can solve a variety of your business challenges by just asking Watson, Einstein, Siri, Alexa, HAL (click for the iconic HAL 9000 scene in Space Odyssey) or other “humanizing” names.
In fact Gartner’s 2016 Emerging Technologies Hype Cycle has Machine Learning at the very “Peak of Inflated Expectations.” Those who are familiar with the cycle, know what is likely to come next.
If you want to protect yourself from the hype, here are 3 critical ingredients for your consideration:
At Reltio we’ve been articulating a vision which includes a pragmatic perspective of machine learning (ML) for over 3 years now. Realizing that not only does ML not offer a silver bullet, but there is still much to learn (pun intended) as to how such technologies can ultimately benefit both IT and business. Noted Big Data expert Bernard Marr provides a nice list of use cases that might be applied to your specific business and industry. They key is that a focused set of benefits for each users’ role, must be defined in order for it to be accurately measured so it doesn’t get labelled yet another (data) science project with limited value.
#1 CREATE A RELIABLE DATA FOUNDATION
Most companies are NOT ready for any form of AI, ML, or Cognitive Computing to help their business user, because their data is such poor shape to even attempt such an endeavor. Ironically a great IT use case is to use ML to first help improve the consistency, accuracy, and manageability for better data quality (DQ), uncovering patterns, anomaly detection, and assisting humans such as data stewards, to make their job more focused and efficient. Master Data Management (MDM) is a key part of that strategy.
#2 BRING ANALYTICS AND MACHINE LEARNING TO THE DATA
Just as the process of aggregating data to perform historical or predictive analytics is a cumbersome and expensive process. Gathering and blending all of the right data that will guarantee machine learning is effective must be the in the DNA of any Modern Data Management Platform as a Service (PaaS). And in fact it forms the foundation for the Self-Learning Enterprise.
Bolting on AI or ML into legacy master data management (MDM) systems, or using such MDM tools to feed downstream disparate ML tools is putting lipstick on hosted managed services disguised as cloud. Reliable data, relevant insights and recommended actions via machine learning needs to be seamlessly combined into one, single multi-tenant cloud platform, architected from the ground up, for both analytical intelligence and operational execution, through data-driven applications.
Successful execution requires a closed loop of all data, insights, and actions, to ensure accurate metrication for continuously improved outcomes. Further a multi-tenant cloud environment is the only way sufficient storage and processing capacity can be elastically accessed on demand to meet any business need.
Another benefit of a multi-tenant cloud PaaS is the potential to use a wide variety of anonymized data to help with machine learning across all industries. Having a large enough set of data is a critical factor for smaller companies to benefit from the right recommended actions, for common industry use cases.
#3 DON’T GO ALL IN ON ONE VENDOR
In a rush to market that “our tools do it too” large vendors will unfortunately over promise, and under deliver. It’s not their fault, as they must respond to the market, but many face an unenviable task of achieving ingredient #2 above, let alone attempting to now also execute on a plan to deliver their own AI technologies.
An open ecosystem that allows you to choose and partner with the technologies, and domain experts of your choice is critical to getting the most out of a still young and evolving landscape. Most companies are already trying to evolve out of their legacy MDM platforms. Getting further locked into a single vendor delivering both MDM and ML, through siloed disparate tools will not provide “clarity”, and may further complicate an already fragmented data management strategy.
At Reltio, we formed strategic partnerships with companies like QuintilesIMS to combine our strengths to jointly deliver on a vision for the next generation MDM and analytics capabilities for life sciences.
In summary, look to master your data in #1 for a reliable data foundation. #2 ensure that it covers all data types, sources, and modes of consumption in a seamless feedback loop on a modern data management platform architected from the ground-up to avoid further siloing your data. Finally, #3 give yourself the openness and flexibility of your partners of choice to meet your business needs.
You don’t want a HAL-like failure that prevents you from realizing your true goal of improving your business.